2022–2023 CFP
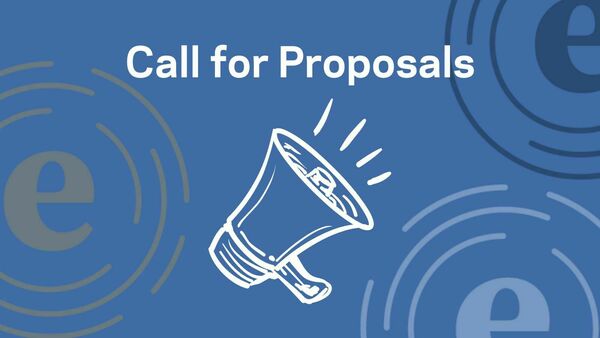
Each year, the Lab releases a Call for Proposals (CFP) for the purpose of funding practical and applied interdisciplinary research in tech ethics.
The focus of the 2022–2023 CFP is “Auditing AI.”
Information about the award winners is included below. Final deliverables—e.g., training modules, proof-of-concept-tools, audit frameworks, etc.—will be accessible through the Lab’s website after they have been completed.
*Note: While the application period for the 2022–2023 CFP has closed, you can refer to the application packet that was used for more information about the CFP process.
Award Winners
AI Audits for Whom? A Community-centric Approach to Rebuilding Public Trust in Singapore
Awardees: Jason Grant Allen (Singapore Management University), Sharanya Shanmugam (Singapore Management University), Zhang Wenxi (Singapore Management University)
The governance of artificial intelligence (AI) to mitigate societal and individual harm through ethics-by-design calls for equal attention to responsible data use before public trust can be conferred to AI technologies. Since trust is fundamentally rooted in community relationships, AI regulators seeking public acceptance toward AI innovation must attend to community-centric pathways to integrate data subjects’ voices in AI ethical decision-making. While traditional actuarial methods in financial audits can indicate a diverse range of evidence used to determine legal compliance, the researchers suggest that community interests and data subjects’ voices should not be absent in AI audit models. This research proposal will explore Singaporean (and Asian) perspectives on AI regulation to inform the motivations for using AI audits to rebuild public trust. Research analysis on the proposed scope and methodologies of AI audits will be followed by recommendations on the relevant skillsets for future AI auditors.
Algorithm Auditing, The Book
Awardee: Christian Sandvig (University of Michigan)
Would-be algorithm auditors presently have little guidance to begin learning about their research method. This project proposes to use an experimental writing process—the “book sprint”—to produce a short book about algorithm auditing. The book would be written by successful auditors and their legal advisors in a concise, accessible style, and published by a respected press. As a crossover title it would aim at both potential auditors (including algorithm designers, investigative journalists, and academic researchers) and others who wish to understand this area (including policymakers, regulators, and the general public). It will be grounded in the longstanding social scientific audit study literature, also known as correspondence studies or paired testing, giving it a distinctive voice. These foundational ideas will be updated and applied to contemporary systems by leading researchers in computing, employing real-world examples from cutting-edge contemporary audits.
Audit4SG: Toward an Ontology of AI Auditing for a Web Tool to Generate Customizable AI Auditing Methodologies for AI4SG
Awardees: Cheshta Arora (Independent Researcher), Debarun Sarkar (Independent Researcher)
This project aims to develop an ontology of AI auditing, which will be used to build an auditing web tool. The target users of the tool are external AI auditors of AI4SG (AI for Social Good), who will be able to generate customizable AI auditing methodologies. Along with the custom AI auditing methodology generated by the user, the tool will provide the user with a report card noting the pros and cons of the chosen AI auditing methodology. The web tool will be a proof of concept whose underlying ontology will contribute to the field of relational ethics in AI auditing that can account for diverse interests, multi-directional processes, multi-scalar networks of actors, institutions, data, algorithms, infrastructure, values, and knowledges. For heuristic purposes and based on the team’s domain expertise, the project will limit itself to three domains of AI4SG: economic empowerment, education, and equality and inclusion.
Best Practices for Communicating and Writing AI Audits
Awardee: John Gallagher (University of Illinois Urbana-Champaign)
This project aims to provide AI auditors with the discipline-specific training required to reach multiple expertise levels in their reporting, while navigating potential pitfalls of ambiguity. It will achieve this goal via the analysis and synthesis of one-on-one interviews conducted with 107 machine-learning scientists and researchers (118 interviews, over 86 recorded hours). This dataset, gathered for an unfunded project, contains unanalyzed responses to direct questions about communication with AI scientists, domain experts (non-AI), and the public. This large amount of process-based information requires trained human coders to identify best practices and themes. Drawing upon communication frameworks from the field of writing studies, these findings will be synthesized into training modules and disseminated to the academic community.
Building a Model of Participation of Children, Families, and Communities in AI Audits for Educational Services in Brazil
Awardees: Bruno Bioni (Data Privacy Brasil Research Association), Marina Garrote (Data Privacy Brasil Research Association), Marina Meira (Data Privacy Brasil Research Association), Júlia Mendoça (Data Privacy Brasil Research Association)
This project will concentrate on methodologies for meaningful participation of children, families, and communities in AI audits, focusing on educational technologies in Brazil. Both awareness and tools to promote participation are lacking and needed, given i) the penetration of AI-based solutions in schools in Brazil and ii) the current process to regulate AI nationally, which has so far paid little attention to this issue. The same reasons for concern also reveal the good timing for the project, with a community of technology and children's rights activists and scholars that has become increasingly more engaged in recent years. The second goal is to move forward in building a model for stakeholder participation geared toward this specific public. The scope is justified by a favorable context on three fronts: hits and misses in law-mandated participation models, a robust framework for child protection, and a problematic but timely process of AI regulation. The activities will consist of workshops and qualitative research to produce a roadmap proposal.
A Capability Approach to Ethics-Based Auditing in Medical AI
Awardees: Mark Graves (AI & Faith), Emanuele Ratti (University of Bristol)
Recently, it has been proposed to address the ethical challenges posed by AI tools by taking inspiration from auditing processes. This approach has been called ethics-based auditing (EBA), and it is based on an underlying conception of ethics that significantly draws from the recent principled turn of AI ethics, which is notoriously fraught with difficulties. This project proposes an alternative framework for EBA that is not based on AI principlism. In particular, it aims at conceptualizing EBA on the basis of the capability approach. Rather than checking for compliance to vague principles, EBAs should investigate the impact of AI tools to capabilities. The team formulates a preliminary characterization of capability-based EBA in medical AI. Deliverables will consist of a manuscript delineating the framework, a prototype AI tool that can be used for both internal and external auditing, and a conference paper.
Course Development for EU AI Act Certified Auditors
Awardee: Ryan Carrier (ForHumanity)
Independent Audit of AI Systems (IAAIS) provides a comprehensive risk framework in the same fundamental principles as Independent Financial Audit. ForHumanity is working towards building such an infrastructure of trust by enabling independent, third-party-assured compliance with the law. ForHumanity, a non-profit, public charity, ensures auditor/pre-auditor independence and anti-collusion principles across the ecosystem while training experts who conduct audit/pre-audit services while upholding the Code of Ethics and Professional Conduct. This project proposal is to develop and deliver an online 30-hour ForHumanity Certified Auditor Course (FHCA) on conformity assessment under the EU AI Act, built on the foundations of ForHumanity’s audit criteria.
Development Of An AI Auditing Educational Training Simulation: Use Case Of Ascertaining AI Biases When A Legal Requirement Requires Assessing Machine Learning Models In Automated Employment Decision Tools Apps
Awardee: Lance Eliot (independent researcher)
This proposal describes a research project that entails the development of an AI Auditing educational training simulation, including the creation of an Automated Employment Decision Tool (AEDT) app utilizing Machine Learning (ML) that would be at the core of the simulation training experience. Extensive materials would also be devised. In brief, the research-produced simulation would enable a real-world hands-on approach to learning about AI Auditing and do so in a context involving the rising interest in AI Bias audits in automated employment decision settings such as the New York City (NYC) legal requirements enacted into law. We believe that no such simulation yet exists. This research project will break new ground in providing an educational open-source capability for those interested in being trained in AI Auditing. Also, the simulation can be used as a platform by researchers pursuing the advancement of AI Auditing methodologies, policies, ethics, legal facets, and the like.
Domain-Specific Legal Explainable Artificial Intelligence for AI Auditing
Awardees: Łukasz Górski (University of Warsaw), Shashishekar Ramakrishna (Research Advocacy and Management School of Intellectual Property Rights)
The aim of this project is to study the feasibility of developing a hybrid symbolic/subsymbolic explainable artificial intelligence system for law. The main assumption of this project is that the inclusion of legal domain knowledge in a system, something a symbolic AI excels at, would help to generate explanations that are of high use for the potential users of legal AI systems. In order to achieve this aim, a proof-of-concept system is to be developed and evaluated.
Expanding AI Audits to Include Instruments: Accountability, Measurements, and Data in Motion Capture Technology
Awardees: Abigail Jacobs (University of Michigan), Emanuel Moss (Intel Labs), Mona Sloane (New York University)
“Expanding AI Audits” proposes to develop and extend AI audit frameworks to include hardware and other instruments used to collect data used in AI and other data-driven algorithmic applications. The project extends AI audit frameworks to assess not only the outcomes of an AI system, but also to examine the assumptions on which those systems are based. Doing so enables auditors to assess the validity of an AI system, its appropriateness for use in specific contexts, and the conditions under which such assumptions may fail to produce safe and effective outcomes. The project will examine the use of motion capture technology as a mechanism for data collection and AI development and produce an expanded audit framework for hardware and other mechanisms. This framework will be accompanied by a workshop convening technologists, audit professionals, and regulators to disseminate the framework and findings as well as a series of public events.
A Framework for Auditing Organizations’ Responsible AI Maturity
Awardees: Ravit Dotan (University of Pittsburgh), Ilia Murtazashvili (University of Pittsburgh)
The team is creating a framework to audit the responsible AI maturity of organizations. The framework will evaluate organizations on three aspects: their knowledge of AI ethics, how well they embed AI ethics practices into their workflows, and what oversight structures they use for AI ethics. Beyond an auditing framework, deliverables will include empirically based strategies and tools for increasing organizations’ responsible AI maturity. This framework is a part of a larger project. First, the proposed framework is based on previous work that was done with the support of last year’s Notre Dame-IBM Tech Ethics Lab grant. Second, the framework will be developed in the context of a new lab at the Center for Governance and Markets at the University of Pittsburgh. In addition to developing this framework, the lab will apply it to the local tech ecosystem. Last, the lab will partner with other teams to support similar projects in other regions.
From AI Audit to Accountability: Understanding the Policy Perspectives Required for Accountability
Awardees: Charles Ikem (PolicyLab Africa), Jerry Monwuba (PolicyLab Africa), Kazeem Oguntade (PolicyLab Africa), Gideon Osadolor (PolicyLab Africa), Cornelius Udeh (PolicyLab Africa)
The most horrific tales about algorithmic injustice are tied to pre-mature AI deployments leading to social and benefits injustice. The AI audit ecosystem remains fragmented with tools and frameworks scattered and, in some cases, closed and gatekept. Given the increasingly visible policy developments mandating audits and the proliferation of algorithmic products, algorithmic audits are increasingly critical tools for holding vendors and operators accountable. But without an understanding of the drivers of AI audit relating to a particular sector, it is hard for meaningful policy to drive and hold operators accountable. The researchers propose to map the AI audit ecosystem trends and their relationship with societal and technological innovation and identify policy mechanisms, frameworks, and practical recommendations for policy developments and future research to advance the AI audits for accountability.
A Model AI Audit Process in the Pharmaceutical Industry
Awardees: Nick Bott (Takeda Corporation), John Chan (Takeda Corporation), Dustin Holloway (Takeda Corporation), Alejandra Parra-Orlandoni (Takeda Corporation), Tim Smith (Takeda Corporation)
Auditing methodologies for artificial intelligence (AI) within the life sciences will become essential as AI-driven algorithms and their integral data find new uses across the healthcare value chain, become more impactful in medical decisions, and are subject to more sophisticated regulation. The team aims to leverage Takeda’s experience implementing complex regulatory compliance to design an end-to-end AI audit process that will be generally applicable across the pharmaceutical industry. They will begin by aligning on standard ontologies for AI risk. In parallel, the team will design tools and software applications to embed ethics into the engineering lifecycle. Finally, they will engage a variety of stakeholders and experts to design a complete process map for an end-to-end AI audit that can be practically implemented in a healthcare company.
*Note: Takeda Corporation has elected not to receive a monetary award.
Open Source Audit Tooling (OAT)
Awardee: Briana Vecchione (Cornell University)
Despite growing recognition of the importance of AI audits, current solutions often fall short of auditors’ goals for thorough and accountable evaluation. This is partially due to the fact that the field is early in its development, and many of the basic terms—such as “auditing”—refer to a variety of aims and methods. This work aims to identify existing tools and resources used by auditors when analyzing AI systems by developing a taxonomy, distributing a survey, and hosting rounds of interviews with audit tool developers and/or practitioners about the tools they have developed and used. In doing this, the hope is to illuminate the landscape of existing tools and encourage solutions that allow for rigorous and accountable scrutiny of AI.
Process Audits for AI Bias: A Streamlined Framework for Independent Auditing of Algorithms
Awardees: Shea Brown (BABL AI), Khoa Lam (BABL AI), Benjamin Lange (BABL AI)
Although artificial intelligence (AI) has reshaped humanity in various positive ways, its potential for harm—such as bias—has sparked intense debates about how such technology can be effectively managed and governed. In recent years, independent auditing has been advocated as an accountability mechanism in various legal and industry frameworks, but its effectiveness remains questionable in the absence of consensus on auditing standards. The researchers aim to develop a standard methodology for the bias auditing of algorithmic systems, namely a process audit. They apply this auditing framework to derive audit criteria for an AI bias process audit for the case study of New York City’s Local Law 144, which requires annual independent impartial bias audits for automated employment decision tools starting in January 2023. Lastly, the team deploys audit criteria by conducting bias audits of relevant organizations and evaluates the process audit framework in practice using qualitative methods.
Trauma-Informed AI: Developing and Testing a Practical AI Audit Framework for Use in Social Services
Awardees: Suzanna Fay (The University of Queensland), Philip Gillingham (The University of Queensland), Paul Henman (The University of Queensland), Lyndal Sleep (The University of Queensland)
AI is increasingly being used in the delivery of social services. Offering opportunities for more efficient, effective, and personalized service delivery, AI can also generate greater problems, reinforcing disadvantage, generating trauma, or re-traumatizing service users. Conducted by a multi-disciplinary research team with extensive expertise in the intersection of social services and digital technology, this project seeks to co-design an innovative AI trauma-informed audit framework to assess the extent to which an AI’s decisions may generate new trauma or re-traumatize. It will be road-tested using multiple case studies of AI use in child/family services, DFV services, and social security/welfare payments.
Unpacking Algorithmic Infrastructures: Mapping the Data Supply Chain in the FinTech and Healthcare Industries in India
Awardees: Shweta Mohandas (The Centre for Internet & Society), Amrita Sengupta (The Centre for Internet & Society), Yatharth (The Centre for Internet & Society)
Large-scale adoption of AI systems across different sectors over the last few years has also foregrounded concerns around algorithmic bias, transparency, data privacy, and safety, within an overarching framework of ethics. Mechanisms to develop and deploy ethical AI systems have been a matter of debate, especially in India and across the majority world, primarily given the invisibility of algorithmic infrastructures that underlie the digital economy. Through a study of the data supply chain infrastructure in the financial services and healthcare industries in India, this project will aim to critically analyze the ethical frameworks that are adopted (or lack thereof) to develop and deploy AI systems in these sectors. Based on learnings from the study, it will offer an overview of best practices and an assessment framework that may inform efforts in auditing AI, and help develop robust data management and regulation practices in adherence with global but contextual ethical standards.
Users’ Trust in Human Resource AI Tools and AI Audits: How Can AI Audits Potentially Help Users of Human Resource AI Tools Gain More Trust in the Tools?
Awardee: Tina Lassiter (The University of Texas at Austin)
AI auditing is evolving rapidly and will become increasingly significant. Legislation, government agencies, and private companies are introducing a wide variety of AI audits for various sectors and industries. This study will focus on audits of AI tools for human resource (HR) decisions, particularly hiring decisions. Such decisions are highly consequential. While there has been extensive research done with regard to AI audits in this field in general, the fairness of HR AI tools, and the trust users have in the tools, there has been less focus on how AI audits could specifically increase the trust in such tools. By studying how users (applicants, employees, recruiters, HR managers) feel about different AI auditing models and in particular which parts of audits affect their trust towards AI tools, this study aims to gain deeper insights into the value and problems of AI auditing for users of AI in general.
UX Work as an Auditing Opportunity: Exploring the Role of Conversational UX Designers
Awardee: Elizabeth Rodwell (University of Houston)
User experience (UX) as a field has expanded rapidly, with many companies rushing to hire user experience professionals for the first time, and the intersection of usability and artificial intelligence becoming a significant part of the field. Therefore, UX researchers and designers are in a unique position to contribute to a more accountable AI and to develop tools and processes to audit this technology. This project focuses specifically on UX professionals working within a challenging subfield of conversational AI: voice assistants. It proposes a fieldwork-based collaboration within which the researcher analyzes how social inequities are reproduced in AI and establishes which methodologies UX can contribute to increasing oversight and accountability for businesses. UX has already laid the groundwork to intervene when a company’s products are working against the best interest of its users. But it must go further, in always considering ethics part of the user experience.