Preserving Privacy when Learning Individualized Treatment Rules: Sensitive data can provide valuable insights while still remaining private
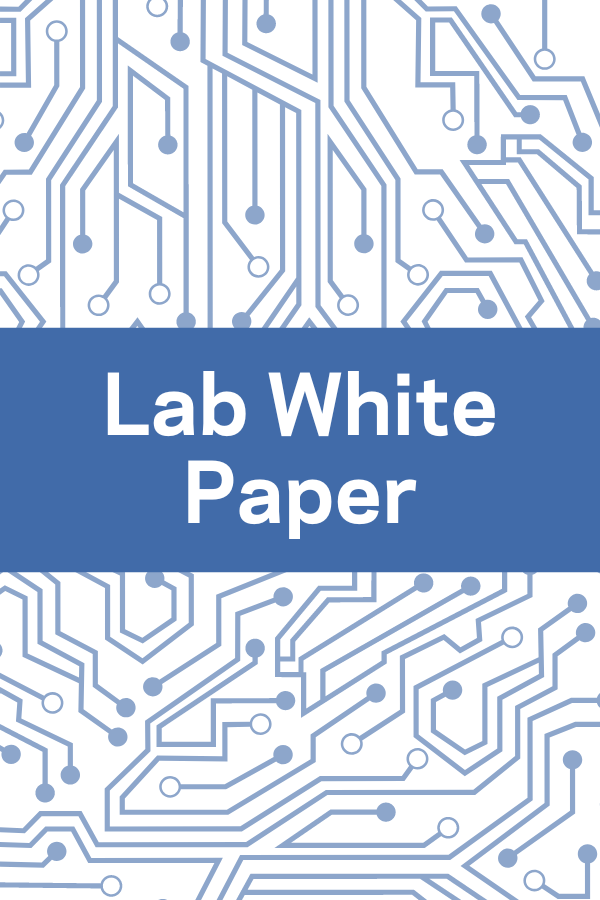
Publication Date: Spring 2023
Abstract
Clinical trials and other medical studies routinely collect sensitive data from individual participants. In many cases, this information is then used to inform individualized treatment rules (ITRs). Outcome weighted learning (OWL) is a machine learning method for developing ITRs in a manner that ensures the best possible treatment benefit. Though beneficial in many ways, the use of OWL (or any other machine learning method for that matter) for fitting a treatment assignment model introduces privacy concerns, as it has been shown that models fitted on sensitive data are susceptible to attacks revealing private information. Differential privacy (DP) is a popular framework for providing mathematically provable privacy guarantees when operating on sensitive data. Though previous work has been devoted to applying DP to related methods, prior to this project it had not previously been applied to OWL, despite the clear reasons for doing so. To apply DP to OWL, we developed a novel algorithm approximating OWL and proved that it satisfies DP. We then assessed the performance of DPOWL via simulation studies. The results demonstrate that it is possible to simultaneously fit an effective OWL model for individualized treatment assignment and provide privacy guarantees to medical study participants.
Why the Healthcare Industry Should Care
Information collected via medical studies, such as clinical trials, typically has a quality unmatched by data obtained from other sources due to the carefully prescribed procedure for collection. As a result, such data should be used whenever possible. Considering methods for using these data with robust privacy guarantees could permit ethical data use in previously unexplored situations.
In today’s data-driven world, the public is more concerned about the privacy of their personal information than ever. Though well-executed clinical trials are extremely beneficial to society, they may also be a source of apprehension for participants regarding the use and protection of their personal data, which could discourage participation. Privacy-preserving analysis methods represent an ethical way to settle these concerns.
By utilizing privacy-preserving counterparts to popular methods of analyzing clinical trial data, researchers can engender confidence in the public that sensitive data is handled appropriately.