Learning Symbolic Models for Interpretability in Healthcare Applications
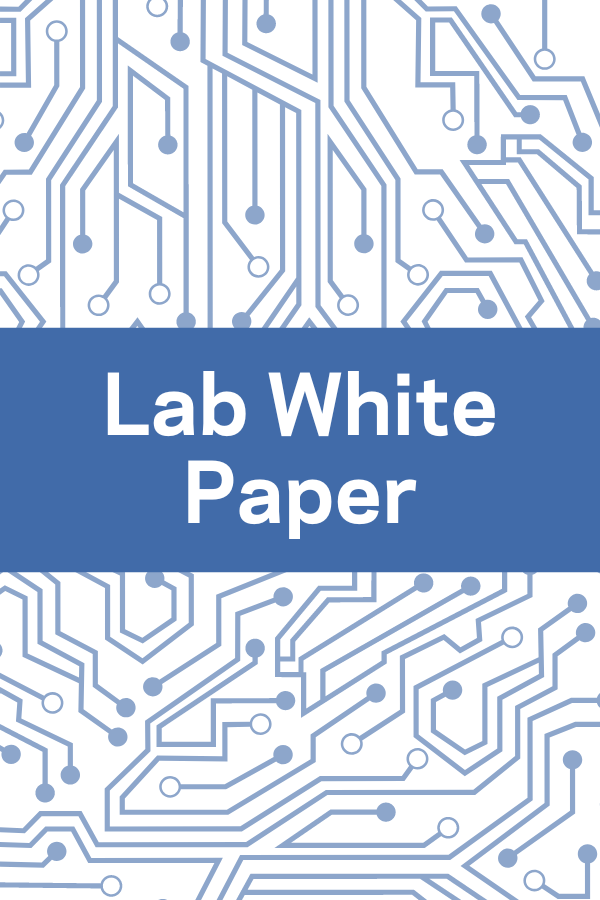
Publication Date: Spring 2023
Abstract
Recent trends in data science research have gravitated toward “black box” modeling approaches, in which the meaningful pathway from input to output is not explicitly understood by human users. Despite impressive predictive performance within a wide array of contexts “black box” models raise difficulties for domain experts to extract the concrete patterns that machine learning algorithms are designed to uncover. In this work, we discuss the potential of symbolic modeling as a more interpretable machine learning approach, especially within high stakes fields, such as healthcare. We suggest the first step toward achieving such a model is discovering the useful building blocks, or structural relationships, that live within a particular dataset. Using simple association measures, we find that ground-truth building blocks can be discovered on synthetic machine learning problems.
Why the Healthcare Sector Should Care
- Symbolic models are represented by explicit mathematical notation that users can work with and analyze using procedures that are typically learned by the end of high school. As a result, the learned models are highly accessible for interpretation and analysis in the clinical setting.
- A concrete understanding of risk patterns will allow clinical staff to design more tailored interventions and procedures to improve patient outcomes.
- Symbolic model representation is highly portable, and extracted patterns can therefore seamlessly transfer to other health applications.
- Interpretable modeling facilitates transparency and accountability to patients and stakeholders due to the traceable nature of predictions.